ベンチマーク条件
繰り返し回数は100回で平均値を計算にかかった時間とする。
ベンチマーク結果
512x512配列のフーリエ変換を16回繰り返すケースや、16x16配列のフーリエ変換を16384回繰り返すケースなど、データサイズを固定した比較。
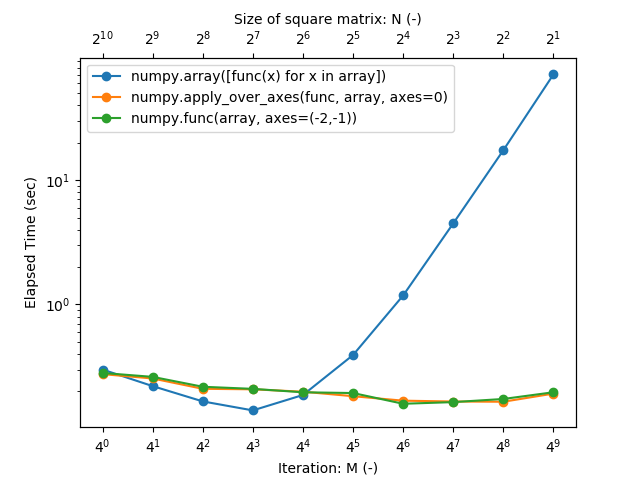
ベンチマークコード
#!/usr/bin/python
import sys
import numpy
import timeit
numpy.set_printoptions(threshold=numpy.inf)
numpy.set_printoptions(linewidth=100)
def func(
x: numpy.ndarray
, *args
, **kwargs
) -> numpy.ndarray:
return numpy.fft.ifft2(
numpy.fft.fft2(
x
, axes=(-2, -1)
)
, axes=(-2, -1)
)
def test0(
a: numpy.ndarray
) -> numpy.ndarray:
return numpy.array(
[
func(
x
)
for x
in a
]
)
def test1(
a: numpy.ndarray
) -> numpy.ndarray:
return numpy.apply_over_axes(
func
, a
, axes=0
)
def test2(
a: numpy.ndarray
) -> numpy.ndarray:
return func(
a
)
loop = int(sys.argv[1])
ai = a0 = numpy.random.rand(
int(sys.argv[2])
, int(sys.argv[3])
, int(sys.argv[4])
)
data = []
while True:
print(loop, numpy.shares_memory(a0,ai), ai.shape)
# b = test0(ai)
# c = test1(ai)
# d = test2(ai)
# print(
# numpy.allclose(b,ai) ,b.size, b.shape, b.dtype
# , numpy.allclose(c,ai) ,c.size, c.shape, c.dtype
# , numpy.allclose(d,ai) ,d.size, d.shape, d.dtype
# )
# do something
result = numpy.asarray(
[
timeit.timeit('test0(ai)', globals=globals(), number=loop)
, timeit.timeit('test1(ai)', globals=globals(), number=loop)
, timeit.timeit('test2(ai)', globals=globals(), number=loop)
]
) / loop
data.append(
numpy.hstack(
(
ai.shape
, result
)
)
)
print(
numpy.hstack(
(
ai.shape
, result
)
)
)
if ai.shape[-1] == 2:
break
ai = a0.reshape(
numpy.array(ai.shape) * numpy.array([4, 1, 1]) // numpy.array([1, 2, 2])
)
data=numpy.asarray(data)
numpy.savetxt(
"test.csv"
, data
, delimiter=','
)
print(data)
sys.exit(0)
ベンチマークプログラム出力
$ python3 numpy-test.py 100 $((1)) $((32*32)) $((32*32))
100 True (1, 1024, 1024)
[1.00000000e+00 1.02400000e+03 1.02400000e+03 2.99823032e-01 2.75467374e-01 2.81458376e-01]
100 True (4, 512, 512)
[4.00000000e+00 5.12000000e+02 5.12000000e+02 2.20620512e-01 2.54105628e-01 2.61754181e-01]
100 True (16, 256, 256)
[1.60000000e+01 2.56000000e+02 2.56000000e+02 1.66212180e-01 2.09874278e-01 2.17961328e-01]
100 True (64, 128, 128)
[ 64. 128. 128. 0.14071824 0.20793606 0.20965693]
100 True (256, 64, 64)
[2.56000000e+02 6.40000000e+01 6.40000000e+01 1.87145058e-01 1.98881604e-01 1.96789085e-01]
100 True (1024, 32, 32)
[1.02400000e+03 3.20000000e+01 3.20000000e+01 3.92263347e-01 1.83027720e-01 1.94043517e-01]
100 True (4096, 16, 16)
[4.09600000e+03 1.60000000e+01 1.60000000e+01 1.18159345e+00 1.68470212e-01 1.59101686e-01]
100 True (16384, 8, 8)
[1.63840000e+04 8.00000000e+00 8.00000000e+00 4.48839465e+00 1.65902573e-01 1.64049939e-01]
100 True (65536, 4, 4)
[6.55360000e+04 4.00000000e+00 4.00000000e+00 1.73932597e+01 1.65182048e-01 1.73866723e-01]
100 True (262144, 2, 2)
[2.62144000e+05 2.00000000e+00 2.00000000e+00 7.07741759e+01 1.92086089e-01 1.96911049e-01]
[[1.00000000e+00 1.02400000e+03 1.02400000e+03 2.99823032e-01 2.75467374e-01 2.81458376e-01]
[4.00000000e+00 5.12000000e+02 5.12000000e+02 2.20620512e-01 2.54105628e-01 2.61754181e-01]
[1.60000000e+01 2.56000000e+02 2.56000000e+02 1.66212180e-01 2.09874278e-01 2.17961328e-01]
[6.40000000e+01 1.28000000e+02 1.28000000e+02 1.40718243e-01 2.07936061e-01 2.09656926e-01]
[2.56000000e+02 6.40000000e+01 6.40000000e+01 1.87145058e-01 1.98881604e-01 1.96789085e-01]
[1.02400000e+03 3.20000000e+01 3.20000000e+01 3.92263347e-01 1.83027720e-01 1.94043517e-01]
[4.09600000e+03 1.60000000e+01 1.60000000e+01 1.18159345e+00 1.68470212e-01 1.59101686e-01]
[1.63840000e+04 8.00000000e+00 8.00000000e+00 4.48839465e+00 1.65902573e-01 1.64049939e-01]
[6.55360000e+04 4.00000000e+00 4.00000000e+00 1.73932597e+01 1.65182048e-01 1.73866723e-01]
[2.62144000e+05 2.00000000e+00 2.00000000e+00 7.07741759e+01 1.92086089e-01 1.96911049e-01]]